10 March 2025
Generative AI is at the heart of current technological innovations. Powered by giants like OpenAI, it promises incredible results while raising fundamental questions: How do these engines actually work? Are they as smart as we think they are? And above all, how far can we go in using them?
During the conference on generative AI, on Tuesday, September 17, 2024 in the plenary room of the show Paris Retail Week, @Iban Touchet, director of @Incremys, provided enlightening answers to these questions, by addressing in depth the How AI engines work, the importance of data quality while revealing concrete application cases that are working today. In this article we explore a detailed account of these crucial points.
Understanding how AI engines work
The generative AI engine is based on billions of parameters adjusted during its training from huge databases. Contrary to what one might imagine, these are not machines capable of understanding or reasoning. The approach used, while powerful, is fundamentally probabilistic.
Early AI engines were mainly used for simple image recognition tasks. For example, an engine could learn to tell a dog from a cat after being exposed to millions of images of these animals. He then “guessed” the animal presented by adjusting its internal parameters according to what he had learned.
For text, the task is much more complex. AI models generate the most words Likely based on the input text. If two different people had to guess the rest of the same sentence, they could have completely different suggestions. This reflects the non-deterministic nature of language, making the textual prediction task more uncertain.

Technological implications
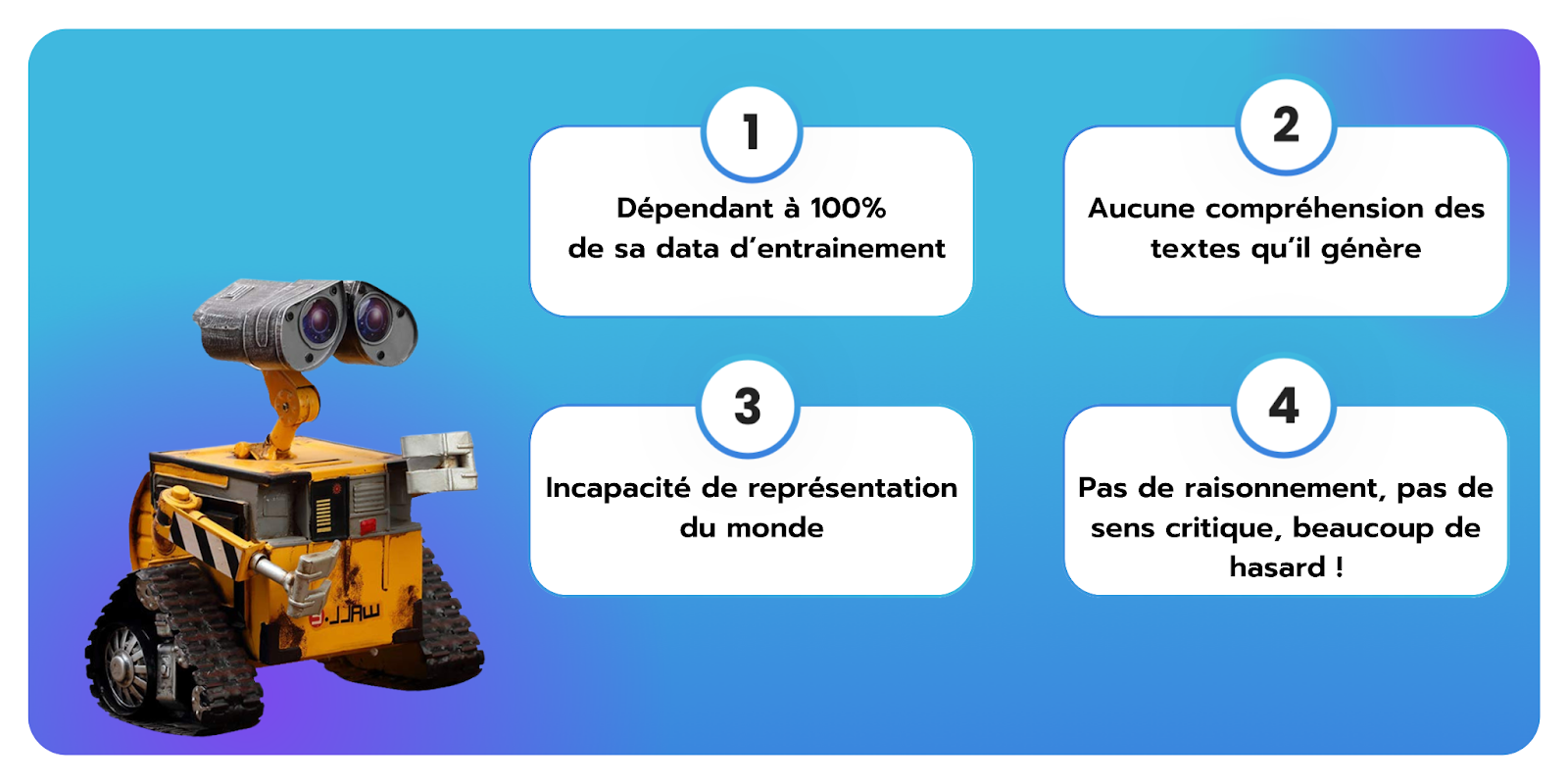
Dependent 100% on your training data
AI engines are 100% dependent on the data provided to them. For example, if an AI is trained on pro-democratic texts, it will produce results that are aligned with that ideology. Likewise, if it is nourished by dictatorial texts, it will generate responses favorable to this regime.
No understanding of the texts it generates
The AI generates bits of words one after the other without any understanding of the overall meaning of the sentence once finished.
Inability to represent the world
AI can't understand the physical or emotional world like humans can. Concrete concepts such as the 5 senses (see, touch, smell, smell, hear, taste) or feelings (joy, sadness, jealousy) are completely absent from its functioning. Can we understand the world by only reading books and without any contact with reality?
No reasoning, no critical sense, a lot of chance!
AI is a technology that generates text or images without real understanding or reasoning, simply sequencing predictions based on the data it has learned.
How tokens work in AI: video demonstration
Contrary to what one might think, AI does not reason in a linear or logical way. It analyzes the probabilities of each token (or piece of word) to predict the most plausible sequence in a given sentence.
In this video from 2022 just before the launch of Sparkneo, we highlight what's going on behind the machine. At the time, we could activate an option in the OpenAI interface to visualize how the probabilities associated with each token generated by the AI worked. This demonstration illustrates the creation process in small successive iterations.
Example of Gpt-4o “reasoning”
Let's illustrate this limitation by using the example of a question asked to GPT-4:
Charlotte has 3 sisters and 1 brother. How many sisters does Charlotte's brother have?
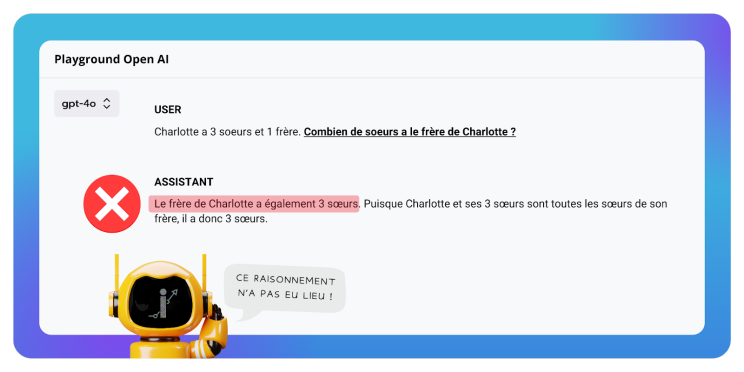
The gpt-4o model, although impressive on the surface, generates an erroneous response (yes, because we hope you have understood that the answer here is 4, Charlotte also being one of the sisters). This example shows that AI has no understanding of family relationships or human logic; it is based on word probability statistics rather than real reasoning. It should also be noted that the sentences generated by the assistant are unnatural or even incomprehensible and above all do not reflect any serious reasoning that a human would have made.
AI is its data
The central element that determines the success or failure of an AI lies in his data. No matter how sophisticated an AI engine is, it can only produce relevant results that match the quality of the information it ingests. An AI can generate surprising, sometimes even absurd, errors if its input data is incomplete, obsolete, or poorly structured.
So the question is not simply to know how to make an AI work, but to understand How the quality of the input data influences each output generated by the engine. In the same way that a machine built to produce high-precision parts will fail if it is fed with faulty materials, an AI fed with imperfect data produces distorted, unreliable, or even inconsistent results.
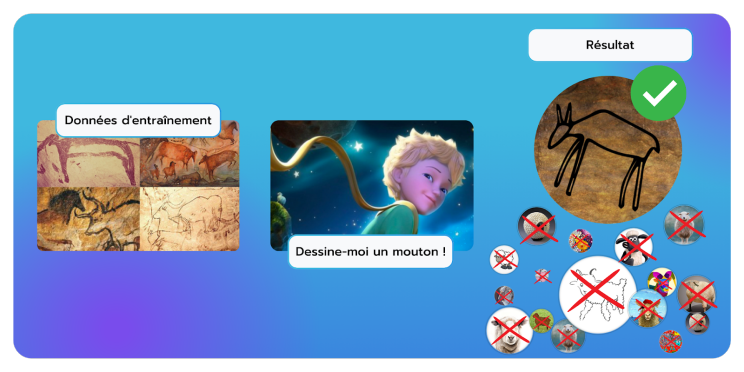
To illustrate this point, here is an analogy with the famous Little Prince tale:
Imagine feeding an AI model with all the prehistoric drawings of caves around the world. If the Little Prince arrived then to ask this strange machine:”Draw me a sheep“, what would it produce?
Unable to design a sheep as we visualize it today, the AI would generate an image based solely on the prehistoric references at its disposal. Perhaps an animal vaguely similar to a sheep, but far removed from what the Little Prince would expect. Why? Because AI is locked into the limits of the data it has been trained to process.
Bad data + AI = hassle
This point is illustrated with an example from real experience: when buying a Wi-Fi repeater on Amazon, we came across a description that the product could also be used as a... Pressure cooker !
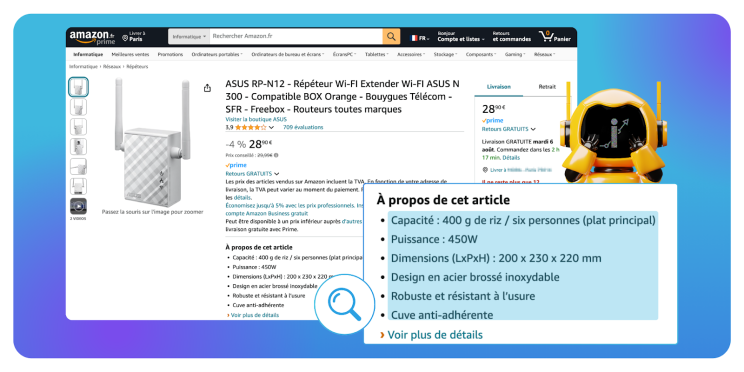
If bad data is used to generate text, the AI will produce an incorrect result that could have disastrous consequences. Because she has no understanding of the real world. That is why it is crucial to fully understand and manage training data before embarking on the use of AI.
Let's imagine that the wrong data for this product is fed to an AI model such as Gpt-4o to generate a product description. The AI would then incorporate this incorrect information into the description without being aware of its absurdity. So, the model could create text claiming that the Wi-Fi repeater is ideal for improving network coverage, and that it would also be... perfect for cooking rice for several people.
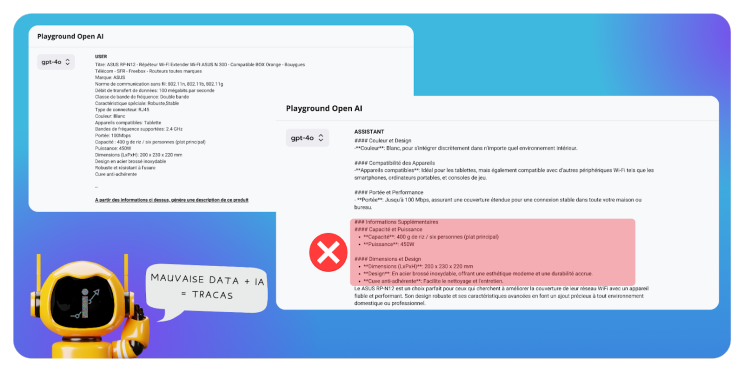
The problem: does all data have a... value?
The fundamental question raised at this conference concerns value of data.
Contrary to initial intuition, All data has no value ! It is tempting to believe that it is enough to accumulate a large amount of data to obtain convincing results, but this approach can be misleading and even dangerous.
Our experience in generating content on a large scale over the past several years has allowed us to distinguish 3 main data families that require different treatments:
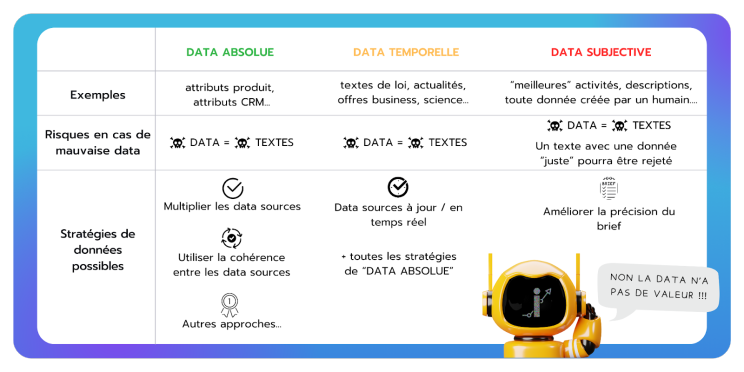
The absolute data
It is unchangeable data that has a intrinsic and fixed value. Examples include product attributes (such as the color of an object) or personal information (such as a birth date). Absolute data does not change over time and is considered reliable. A white product will remain white, and a person born in 2000 will never have a different birth date. In this case, the AI can easily work with this data, as it does not change.
If the absolute data is not filled in, it leads to clear and distinct errors. This typically repeats the example seen above on the Amazon Wi-Fi repeater, whose description incorrectly mentions pressure cooker capabilities. This poor absolute data has resulted in content that is absurd and potentially misleading for users.
To avoid this type of error, it is necessary to set up a data strategy, for example multiplying data sources and compare the information to identify possible inconsistencies.
Temporal data
This data is information whose value is true to a specific moment. This type of data includes, for example, laws, news, knowledge of science but also any business offer because your organization evolves every day.
If AI uses data obsolete in time, it will produce results that are not adapted to current reality. In a commercial context, if an AI is used to create product sheets based on expired offers, it could generate misleading information. Another risk, in a legal framework, would be that AI refers to outdated legal texts, which could lead to serious and even costly mistakes for a company.
To overcome this, it is necessary to set up both absolute data strategies and to add regular refresh process. This means ensuring that the AI always has access to the most current version of the data.
In an organization, time data refers to the relevance of the files available: some are up-to-date with the information while others are outdated and should not be taken into account. So the temporal data concerns much more data than it seems at first glance.
Subjective data
This is data based on human perceptions, which vary from person to person. For example, if you ask several people to describe the “best things to do in Paris”, everyone will have a different answer depending on their tastes and experiences. AI, not being able to judge or understand these nuances, will generate results that may be rejected based on the subjective expectations of the customer.
The risk associated with this type of data is that AI generates content that is”proper“from the point of view of the veracity of the facts stated, but which does not correspond to the expectations of the customer. These discrepancies can cause the generated content to be rejected, even if the basic information is factually correct.
To manage subjective data, the Accuracy of briefs is becoming crucial. It is essential to clearly define expectations before even launching a project with AI. The more specific the brief, the more AI can generate content that meets the specific expectations of the customer.
In an organization, subjective data corresponds to any document produced by a collaborator. Thus, when the same subject is treated by business, marketing and operational teams in separate files, these contents, which nevertheless address the same theme, will lack coherence and alignment between them.
To summarize, No data has no value! Whether for absolute, temporal or subjective data, it is imperative to set up a rigorous data strategy in order to maximize the effectiveness of AI. This includes checking sources regularly, updating information constantly, and clearly setting expectations. In the absence of these measures, AI, as powerful as it is, can only amplify the errors in the data provided to it.
The false marketing promise of generative AI
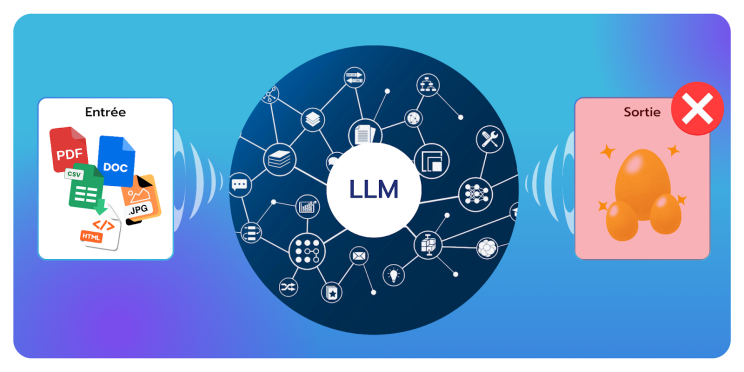
One of the recurring promises in the world of marketing is that the implementation of an LLM engine trained on all of a company's data will make it possible to automate complex tasks and produce results in simply clicking on a button.
This promise seems to us to be greatly exaggerated and difficult, if not impossible, to materialize technologically.
Why? Simply because of data problems. AI is not designed to understand the world critically. She is simply repeating the patterns she learned from the data she was given. As at the level of an organization the data will be contradictory or incomplete, AI will generate results that are just as inconsistent. Moreover, it is unable to distinguish between relevant data and data that is outdated or erroneous. In reality, managing data, especially when it is numerous and varied, requires a complex strategy.
Concrete cases of application of generative AI
Far from marketing promises, generative AI has proven its usefulness in well-defined areas where it has a tangible impact. It offers an automation capacity that, when properly exploited, makes it possible to accelerate processes that were once laborious. However, it is not the magic of a click that is revolutionizing these areas, but strategic integration, based on specific use cases.
Through concrete examples, we will see how, under the right conditions, generative AI can truly transform large-scale content production while highlighting the limitations that are crucial to understand before launching.
The ambition driven by Incremys and Sparkneo
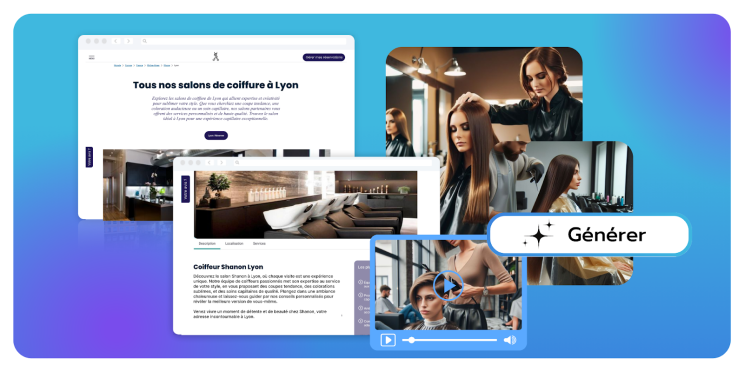
Recall that the ambition of the solutions Incremys and Sparkneo is to allow businesses to generate content on a large scale in a fully automated manner. The aim is to produce publishable texts, images and videos without the need for human retouching. This could, for example, allow a chain of hairdressers to create local campaigns (text, image, video) for each city, while respecting brand consistency...
Texts, images and videos: what technologies are available in a B2B context?
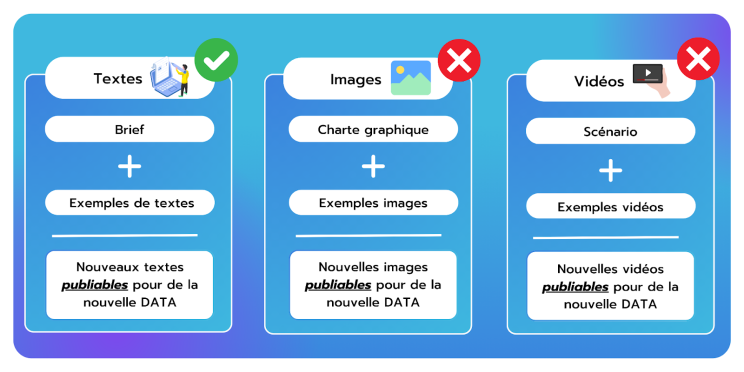
Today, AIs can already generate texts based on predefined briefs, brand DNA, and sample content. However, we recall that the quality of the text will depend directly on the quality of the data provided.
On the other hand, when it comes to the creation ofpictures Or of videos, technology is not yet up to the point where it can fully replace manual work, especially in demanding contexts. While AIs can generate images, they are far from being able to create visuals that are perfectly in accordance with an existing graphic charter on a large scale. The results are often random and require significant human intervention to ensure consistency and quality. As for videos, the idea of generating a movie or a coherent sequence from a scenario is still beyond the reach of current technologies. While AI can help automate certain tasks in video production, such as editing or subtitling, it cannot currently replace a complete video creation process.
AI requires a use-by-use approach with sufficient volume to justify fixed costs.
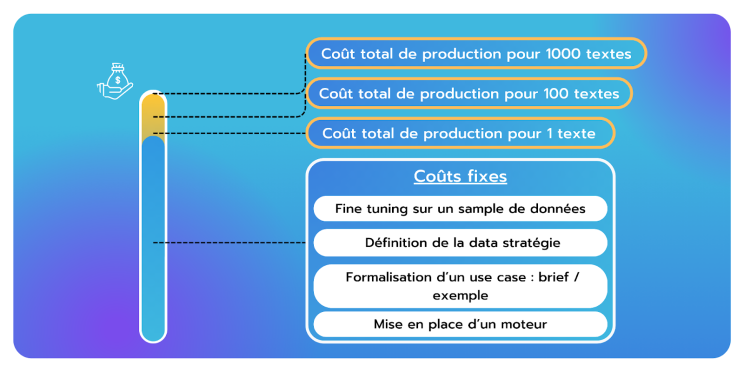
One of the underrated aspects in the adoption of generative AI is its implementation cost. Contrary to the idea that AI could be a simple and inexpensive solution, the reality is that these technologies create fixed costs relatively high, linked to the customization of models, the formalization of a brief, the definition of the data strategy, and fine-tuning to meet specific needs.
This is why, to justify these fixed costs, AI must be deployed in contexts where it can deal with large volumes of data or produce content on a large scale. In other words, investing in AI is only really profitable when a sufficient volume is present.
It is essential to be well Define use cases and to ensure that the Volumetry of the content to be generated is sufficient to guarantee a positive return on investment. Generative AI is therefore particularly suited to sectors that process massive amounts of data or that require repeated and regular production, such as e-commerce, online services or companies with a vast catalog of products.
Application cases that work: 3 families of uses generate volume
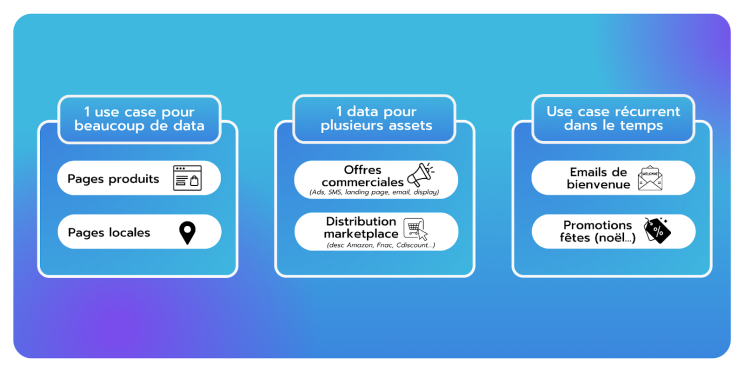
It exists three types of use cases where generative AI can be particularly effective:
1 use case for a lot of data
For example, the generation of product pages for an e-commerce site or local pages. It is interesting to invest in the formalization of a brief because AI will be able to create thousands of pages in a few clicks, which ensures a return on investment.
1 data for several assets
For example, the same commercial offer may be available in different media (advertising, e-mail, SMS, etc.). AI can help automate this combination, making it easier to create marketing campaigns. Again, volume is triggered to absorb fixed costs.
Recurring use case over time
Recurring texts, such as welcome or reminder emails, or seasonal promotions, can also be automated using AI. The client will not create thousands of texts at once, but the temporal recurrence ensures the volume in the medium/long term.
These three families of uses show that generative AI is not a one-size-fits-all solution, but that it can become a powerful asset when applied in specific cases. By optimizing these recurring or large-scale processes, businesses can not only gain in productivity, but also in responsiveness, while considerably reducing their production costs. The key lies in identifying these specific use cases and in implementing a data management strategy that allows AI to shine in these scenarios.
When generative AI is approached in the right way, the results are impressive!
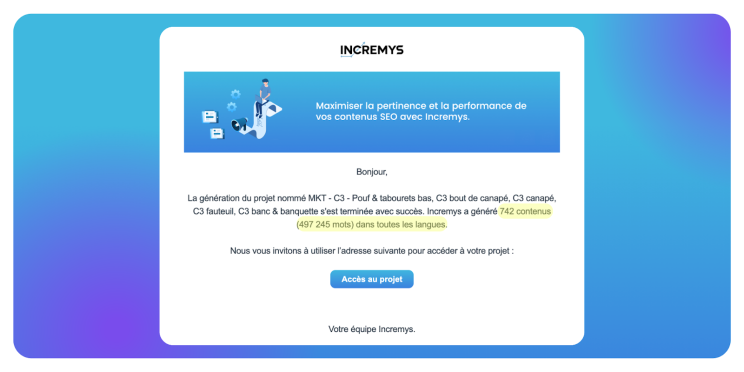
While generative AI presents challenges and requires a methodical approach, it can produce remarkable results when used strategically, with clear goals, and sufficient volume. At this conference, we shared a striking example of an @Incremys client that generated nearly 500,000 words In a few clicks.
Thanks to the use of an AI model specially configured for his project, this client was able to produce 742 pages products, with an average of 740 words per page. This shows how AI can transform the way businesses approach content creation at scale. Instead of spending weeks manually writing hundreds of pages, AI made it possible to complete this task in record time, while respecting the specific criteria established during the initial brief.
3 key things to remember
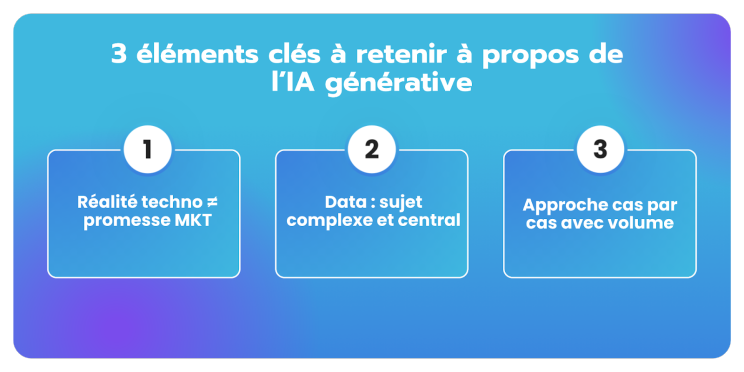
To summarize, it is essential to keep in mind three fundamental points for any business or organization that wants to integrate generative AI:
Technological reality ward off the marketing promise
Although generative AI offers undeniable potential, it is crucial not to get carried away by the often exaggerated promises of marketing speeches. AI, while powerful, has significant limitations, especially in terms of understanding and creating independently. It is therefore necessary to have a realistic vision of what this technology can achieve.
Data: a complex and central subject
The quality of the results of an AI depends directly on the data that is provided to it. Incorrect, outdated, or poorly structured data can lead to inconsistent and even dangerous results. A case-by-case data management strategy is therefore essential to take advantage of AI effectively and avoid costly mistakes.
A case-by-case approach, with sufficient volume
Generative AI does not apply universally to all use cases. For it to be profitable and effective, it is necessary to deploy it in specific contexts with sufficient volume to justify fixed costs. This involves thoughtful thinking about business needs and how AI can meet them, while generating significant savings in terms of time and resources.
Conclusion: There is no easy AI
In conclusion, although generative AI is a powerful technology, it is still limited by the quality and management of the data that feeds it. To take advantage of its capabilities, it is essential to identify the right use cases and then to establish a rigorous data strategy. Marketing promises can be appealing, but don't underestimate the complexity and costs involved in implementing AI-based solutions.
Today, we are seeing a significant acceleration in the use of AI by all our customers in order to save time in creating content. A lot of brands trust Incremys and Sparkneo to support them on their automation challenges using generative AI.
Feel free to get in touch with our team to discuss how our custom AI can specifically meet your needs.
Concrete example